Driving Digital Transformation: The Crucial Elements of Data Pipelines and Governance

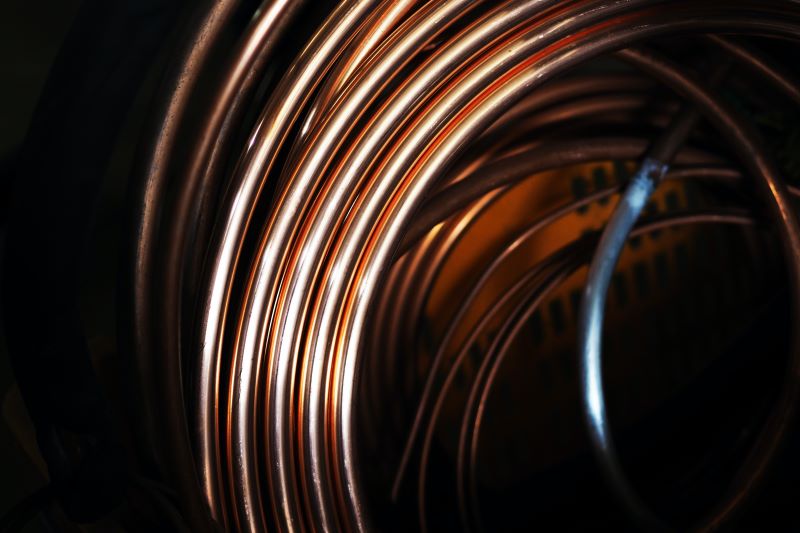
From IC Insider Red Hat
Michael Epley, Chief Architect at Red Hat
Frank La Vigne, Data Cloud Services Global GTM Leader at Red Hat
Data is the lifeblood of the intelligence community. In the modern digital information age, we are deluged by an increasing volume and diversity of data. Finding the most significant parts or link information to convert this raw data into insights that drive policy and action is more challenging than ever. Maximizing the exploitation of this data with artificial intelligence (AI), data science, and decision-making processes is the fundamental challenge of the intelligence community. The significance of both data pipelines and data governance has become paramount as a result. These two concepts collectively help achieve data quality, security, and efficient utilization.
Understanding Data Pipelines
Data pipelines act as the nerves of the data ecosystem – moving the raw bits of information from the sensory cells in the skin to the brain. The action is like an assembly line for this information, and they are a series of steps designed to ingest, clean, transform, model, and visualize data. And like an assembly line, pipelines streamline data management, ensuring a smooth data flow from one phase to the next.
Figure 1: An example data pipeline built with open-source and Red Hat technologies
Whether it’s data science or AI, data pipelines play a critical role, from facilitating feature engineering in machine learning to automating the training and validation of models. Key benefits of data pipelines include:
- Efficiency: Automated data management accelerates processes and minimizes manual errors. Many data pipeline tools encode these processes in ways that can be configuration-managed and allow branching, experimentation, and reuse.
- Data Integrity: Consistent operations across all data ensure reliable analysis outcomes. Pipelines can be wired with validation checks or assertions to ensure they are working as expected and the data being processed is as expected.
- Collaboration: Unified data processing steps enable seamless teamwork among professionals. Data processing is reproducible, sharable, and consistent over time and across teams, even if the data itself is not.
- Scalability: As data grows, pipelines can be expanded or modified to handle more information and integrate new data sources. Pipelines can produce and consume intermediate data and allow users to create composite
Implementing data pipelines is not easy and can present various challenges:
- Shaping: Not all downstream users need the same data in the same way, making the reuse of data pipelines complicated and leading to stovepiping and siloing of the pipelines and the resulting data.
- Scaling to data: Expanding pipelines is easy, but scaling the backend data systems they leverage is complicated – and single or aggregations of pipelines may exceed the capacity of individual backends.
- Provence: By design, they transform and change the data and its representation – but keeping track of how and why is critical when we need to explain downstream conclusions to check for or correct for biases and contamination and give ourselves confidence in our conclusions.
The Imperative of Data Governance
Meeting these challenges is why data governance is imperative. While data pipelines focus on processing, data governance centers on protection and regulation. Policies, processes, and practices help ensure data remains accurate, consistent, and secure, and data governance applies these to our data pipelines to allow us to realize the benefits while addressing the challenges.
Data governance becomes indispensable because of the following:
- Explosive Data Growth: There is a surge in the number and diversity of data sources, including specialized organic sensor platforms, 3rd party IoT devices, social media harvesting, commercial and open-source data collection, and many others, each with their own data types, formats, operational and logistical constraints. A sturdy governance framework maintains data relevance by matching the needs for the data processing to the volume present and resources available.
- Compliance: Governance develops confidence that pipelines protect data from taint, misuse, spills, or exfiltration by attackers or insiders. When automated, we can further reduce risk by asserting where the data was used or manipulated, how, and what information protections were in place. Governance of pipelines can be used to ensure downstream users see only appropriately filtered and processed data and remove potentially malicious data.
- Quality Decision Making: Reliable data analytics and AI-driven insights are contingent upon high-quality data, ensured through governance. Downstream uses of the data – like data scientists who are building or training models – have to know the data is the best available, and good data governance provides this assurance. By applying governance to data pipelines, we can ensure necessary preparation, cleaning, and shaping operations are used and fit the end user’s needs.
- Data Security: Pipelines allow us to separate concerns and roles about how to massage data and who actually gets to access the data. Data governance lets data scientists focus on the former without worrying where the data comes from or can require them to use biased/unbiased, test or synthetic data in development or riskier environments.
To enforce effective data governance:
- Establish a team: Comprising members from diverse departments like data science, IT, and policy. Policy teams can express the procedure so IT professionals can encode these using their data governance tools. In contrast, the data scientists can verify they can still do meaningful work within those policies or provide feedback when policies need to be updated.
- Craft policies: Comprehensive rules addressing various aspects, from data quality to security. Encoding these rules sets clear objectives when building and testing pipelines and helps flag and analyze unexpected behavior.
- Adopt tools: Technology can help automate governance processes. When considering data governance, pipelines, and their management can get complex. Tools that remove the toil and provide consistency will remove pain points and bottlenecks, along with the other benefits noted above.
- Monitor constantly: Find your KPIs and use KPIs to measure governance efficacy, recalibrating as needed. As an aid to governance, building and collecting metadata into our pipelines will provide the required feedback to improve continuously. By completing the circle, governance can ensure the metadata collection is injected into the pipelines.
- Promote governance culture: Your key value stakeholders, the mission owners and data stewards, are critical to making sure the value of a robust governance model is understood, and your data scientists, data engineers, and application developers implement the model and assets like data pipelines to this target.
As we advance in the digital age, efficient data management and robust protection mechanisms become crucial. Data pipelines optimize data utilization, turning it into actionable insights, while data governance ensures the sanctity and security of this data. Together, they form the backbone of a successful data-driven enterprise, highlighting the intertwined relationship between processing efficiency and protective measures in the data domain. Visit red.ht/icn to learn more about this and other issues within the intelligence community.
About Red Hat
Red Hat is the world’s leading provider of enterprise open source software solutions, using a community-powered approach to deliver reliable and high-performing Linux, hybrid cloud, container, and Kubernetes technologies. Red Hat helps customers develop cloud-native applications, integrate existing and new IT applications, and automate and manage complex environments. A trusted adviser to the Fortune 500, Red Hat provides award-winning support, training, and consulting services that bring the benefits of open innovation to any industry. Red Hat is a connective hub in a global network of enterprises, partners, and communities, helping organizations grow, transform, and prepare for the digital future.
About IC Insiders
IC Insiders is a special sponsored feature that provides deep-dive analysis, interviews with IC leaders, perspective from industry experts, and more. Learn how your company can become an IC Insider.
SOURCES
Merger of https://www.linkedin.com/pulse/data-governance-essential-framework-modern-business-success-la-vigne/ and https://www.linkedin.com/pulse/importance-data-pipelines-ai-frank-la-vigne/
Loosely based on the IC RFIs – ATOM SMASHER and NGA Data Science