Charles River to take part in DARPA Learning with Less Labels program

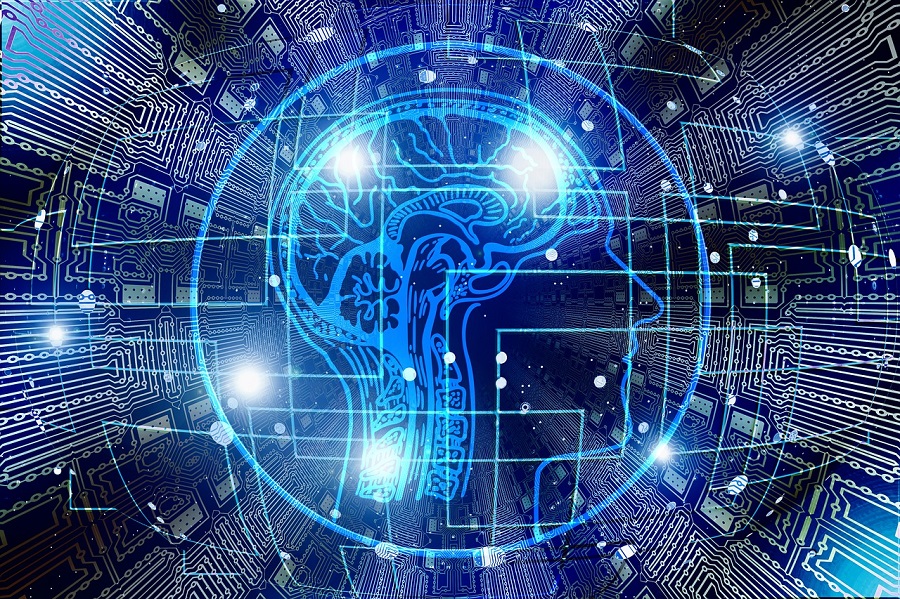
Charles River Analytics Inc. of Cambridge, MA announced on October 29 that it has received funding from the Defense Advanced Research Projects Agency (DARPA) as part of the Learning with Less Labels program. This program is focused on making machine-learning models more efficient and reducing the amount of labeled data required to build models. CRA is partnering with Northeastern University, University of British Columbia, and University of California Irvine to apply its probabilistic programming expertise to learning with fewer labels as part of the Probabilistic Label-Efficient Deep Generative Structures (PLEDGES) approach.
Deep learning models rely on large labeled training sets for accurate results. Unfortunately, labeling data is an expensive, time-consuming process, and the scarcity of labeled training data often results in brittle and incomplete models. Modelers require new approaches to machine learning that can achieve the same performance with fewer labels.
“Current machine learning methods require large numbers of labeled examples to understand the relationships between variables,” explained Dr. Avi Pfeffer, chief scientist at Charles River Analytics and principal investigator on the PLEDGES effort. “These methods may fail to generalize across tasks and to different situations. To address these limitations, we will develop methods to learn the intrinsic properties of the data with probabilistic modeling.”
Probabilistic models are a powerful approach to learning with less labeled data. Structured deep probabilistic models provide neural-like representations that allow comparable performance to state-of-art neural methods while learning from fewer labels. Under the PLEDGES effort, CRA will extend label-efficient semi-supervised learning, model transfer, and active learning to help analysts confidently leverage AI.
Source: Charles River Analytics