The False Promises of AI: How hype has confused our understanding of the future of AI cyberwarfare

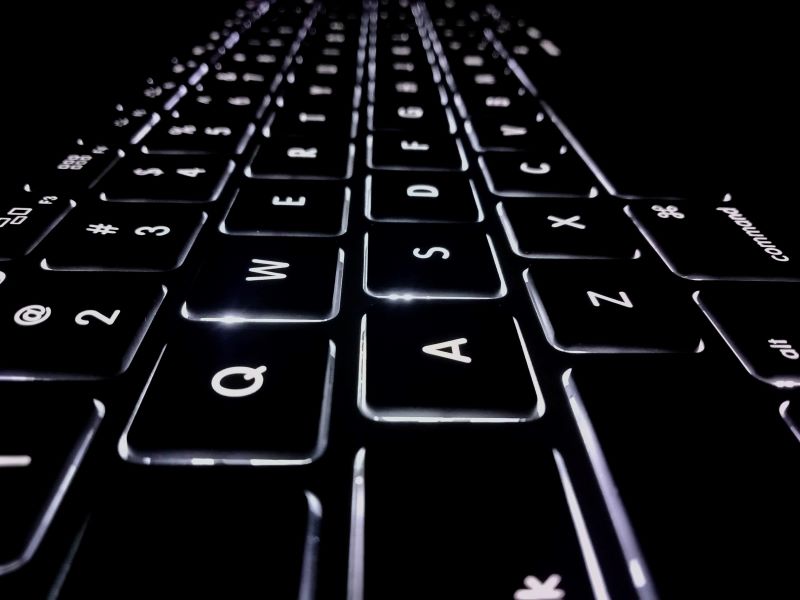
From IC Insider Vectra AI
By Tim Wade, Deputy CTO and Sohrob Kazerounian, AI Research Lead at Vectra AI
“All warfare is based on deception. Hence, when we are able to attack, we must seem unable; when using our forces, we must appear inactive; when we are near, we must make the enemy believe we are far away; when far away, we must make him believe we are near.”
— Sun Tzu, The Art of War
“A computer would deserve to be called intelligent if it could deceive a human into believing that it was human.”
— Alan Turing
The Russia-Ukraine conflict of 2022 wasn’t just waged on a physical battlefield, but a digital one as well. For some organizations, responding to these digital threats involved steps to seek out and remove AI-generated content used to sow disinformation. In the face of the weaponization of AI, there has never been a more important moment to accurately assess the capabilities of today’s AI systems, both in their offensive and defensive uses. This is particularly true after a near decade-long hype cycle in which wide ranging claims have been made about what AI can and cannot do, and even what counts as AI in the first place. For this reason, it is critically important for decision makers to have clear grounding to distinguish what is real AI versus what may just amount to marketing hype or science-fiction.
So, let’s begin by acknowledging what AI can do. First, modern AI, particularly over the last decade or so, has become quite adept at processing large amounts of raw, low-level data, to learn something about its structure, predict something in or about the data, and even generate new data that looks convincingly real, despite having never been seen before. The advances in processing huge amounts of these types of sub-symbolic datasets was made possible by the exponential growth in both our computational resources and the available data from which to train, coupled with the development of deep learning neural network models that take inspiration from the human brain. Deep Learning models have enabled computer vision to perform well at object and facial recognition, have made once unusable speech recognition systems functional and allowed chatbots to converse and answer questions in ways that make it seem as though computers truly understand language.
Furthermore, deep learning is core to the more “advanced” areas of AI where computer agents learn to self-improve according to some reward or objective functions, that instruct them on how to improve performance (e.g., an agent might get more reward for scoring more points in a video game, or winning a game of chess, and therefore increase the likelihood of selecting the behaviors that got it there). This is also true in the now infamous cases of deepfakes, which learn to generate novel images (often of realistic looking, but nevertheless non-existent, human beings) do so by using two competing agents, the first of which tries to fool the other by generating images that look as though they are “real”, while the second tries to guess whether or not the image it is seeing was generated by the first AI, or was actually drawn from the set of real images.
It is important to note that while all the capabilities mentioned so far exist in the set of things AI can do, and all are quite impressive in their own right, they are not without their own shortcomings. For instance, numerous techniques have been devised for creating adversarial examples for computer vision systems, such that imperceptible changes to an image (sometimes even just a single pixel) can result in incorrect predictions about what was in the image. These AI systems simply fail in ways that are different to humans, and often would be considered catastrophic depending on how they are being deployed. The computer vision system on an autonomous car should not classify a school bus as a penguin (for example), simply because a single pixel in the camera image changed values. Furthermore, when reinforcement learning agents are learning to act within an environment, we might use a reward function to help guide its behavior towards an intended goal. But reward functions can often be short circuited, and AI agents can often find ways to maximize their reward that we did not intend in the first place.
To sum up this first category of what AI can do: contemporary AI has developed to the point that it can readily process low-level data at scale, particularly when there are large datasets that exist from which to train and learn. AI currently operates well in domains with massive amounts of data to learn from, and labels and structure that can guide its learning.
At the other end of the extreme exist the cases where AI systems fall short. These cases involve the types of tasks we now typically tend to associate with more advanced intelligence. [Side note: This is no accident. Societal notions of what counts as “intelligent” behavior tend to adapt and change as previously unsolvable problems become solved. Chess, for example, used to represent a clear-cut case where intelligence was a prerequisite to world class performance. Once Deep Blue beat Gary Kasparov however, chess came to be viewed more as a game of brute force].
The problems at this end of the continuum, tend to center around situations that require symbolic and analogical reasoning, and require creativity and world-knowledge. Often, it means being able to perform in arbitrary situations, where large datasets did not previously exist. As an example, consider the Winograd Schemas (a series of tests meant to probe an AI’s intelligence further than the Turing Test which simply probes whether an AI could fool a human into thinking it was a human). The Winograd Schemas include questions that are simple to answer for humans, but would be difficult for AI to strictly deduce from any given language dataset. Questions include things like:
1) The trophy didn’t fit on the shelf because it was too small. What was too small? [The trophy or the shelf?]
2) The trophy didn’t fit on the shelf because it was too big. What was too big? [The Trophy or the shelf ?]
These questions probe something about the natural world that aren’t necessarily going to be explicitly encoded in any Wikipedia page that might get used as training data. There are no websites or texts dedicated to discussing the relative sizing of things that may fit on shelves, compared to the shelves themselves!
For many classes of problems, small datasets might exist but are nowhere near the size sufficient to train the types of deep learning systems common today! In these cases, the spontaneous and creative ability to draw on past experience to solve a particular problem, often from a different domain altogether, becomes the distinguishing factor between what AI is capable of, and what we consider to be the core of human intelligence itself.
Where then, does this leave things with respect to the use of AI in offensive and defensive postures? For now, the primary applications of AI are on the defensive side. Everything from processing large volumes of images and audio, to monitoring huge amounts of (potentially) encrypted traffic, will put to good use the aspects of AI that work well—namely, large amounts of raw data that can be used to train models, without the additional need for logical and creative reasoning.
From the standpoint of cybersecurity, this is obviously a win – but, again, one that must be tempered with a grounded understanding of the expected outcomes. And it isn’t just limited to the traditional enterprise – given the amount of data produced by embedded, ICS, and OT systems is often sufficient to overwhelm the scale at which humans process data there is clearly opportunity for AI to even the score.
There are also the uses of AI on the offensive side, as first mentioned in the at the beginning of this article, and as we predicted a number of years ago. Deepfakes, automated generation of text for spear phishing, and more generally, the automation of very particular types of tasks, will see increasing use by attackers. While the use of AI by attackers is worrying, two things are worth noting.
Firstly, the use of AI in something like creating a deepfake still remains a relatively high-cost activity. As noted by researchers Britt Paris and Joan Donovan, “cheap fakes” (i.e., manipulation of audio-visual data without the use of AI) are as concerning as the use of AI in disinformation, in large part because the ease with which they can be created and deployed. In fact, at the moment of writing, the 5 most recent fact checks (and probably more if one was to look deeper) at AFP on the Ukraine war, all pertain to old images and video, taken out of context in order to create viral disinformation (https://factcheck.afp.com/doc.afp.com.324766G; https://factcheck.afp.com/doc.afp.com.323W3V8; https://factcheck.afp.com/doc.afp.com.324D44M; https://factcheck.afp.com/doc.afp.com.324A9JF; https://factcheck.afp.com/doc.afp.com.324F2GL )
Secondly, while the use of AI for offensive capabilities is in fact real, it is nowhere near Skynet levels of intelligence. There are incentives for certain companies and even individuals, to forward a narrative that AI has reached a level of maturity that it can be used to effectively as an offensive tool. This, however, is all nonsense. What people need to recognize is that AI, while having made impressive gains over the last decade, is still nowhere near the level of intelligence that would be required to allow it to be used autonomously in an offensive context. AI systems are not on the battlefield. AI hackers are not gaining entry into your computer networks. Deceiving ourselves about what AI can and is being used for, will only obscure how we prepare for the real threats we face.
In conclusion, while the situations and application of using “real” AI are expanding, there remain certain specifications to what AI can do. Using and employing AI on the defense is a critical strategy but should be approached with an understanding of what AI is, deployed in a way that leverages tasks machines are explicitly superior to humans at performing. From the offence capability, science-fiction doomsday scenarios of rogue, offensive AI’s aren’t on our immediate horizon, and claims otherwise are primarily a byproduct of marketing and fearmongering. Most importantly, when organizational decision makers are looking at AI, they should really refocus on the outcomes – to do otherwise just invites the charlatans to have a seat at the table.
Explore more on this topic: https://www.vectra.ai/blogpost/ai-two-small-letters-many-big-advantages
About Vectra
Vectra® is a leader in threat detection and response for hybrid and multi-cloud enterprises. The Vectra platform uses AI to detect threats at speed across public cloud, identity, SaaS applications, and data centers. Only Vectra optimizes AI to detect attacker methods—the TTPs at the heart of all attacks—rather than simplistically alerting on “different”. The resulting high-fidelity threat signal and clear context enables security teams to respond to threats sooner and to stop attacks in progress faster—without signatures, decryption or agent installation. Organizations worldwide, including every branch of the federal government, rely on Vectra to detect polymorphic adversaries before they’ve been identified by legacy threat intelligence tools. For more information, visit vectra.ai/federal and contact Michael Wilson, Federal Advanced Programs Group Manager, at mwilson@vectra.ai
About IC Insiders
IC Insiders is a special sponsored feature that provides deep-dive analysis, interviews with IC leaders, perspective from industry experts, and more. Learn how your company can become an IC Insider.